Research
Neuro-inspired AI
Brains are magical. They learn quickly, adapt, evolve, and demonstrate incredible resilience. In our research, we translate insights from the brain into implementations that help machines learn faster, adapt better, potentially evolve, and build resilience.​​
​
One particularly interesting class of substances we’re working with is neuromodulators (such as dopamine and serotonin), which play a key role in the brain's capacity for continuous learning and adaptation. Neuromodulation-driven processes occur across spatio-temporal scales, making both data analysis and modeling challenging. At the WNB Lab, we use various tools to simulate these processes, incorporate them into artificial neural networks, and see if they offer new insights.
​​
Beyond neuromodulation, here’s a list of things that intrigue us (i.e., we’d like to model them!):
-
Neural heterogeneity
-
Glia
-
Neurogenesis
-
(Early) Brain development
-
Evolution
-
Pre-configured brain circuits and architecture​

Brain data analysis
Analysis of brain data is key to interpretation and modeling, and when done properly, can lead to new scientific ideas and experiments, facilitate the development of AI models, and support diagnosis and assessment. The lab collaborates with leading research laboratories around the world and has access to data from a range of organisms, from rodents to primates to humans.
​
Some of the datasets we work with:
-
Human neuroimaging (structural and functional) from healthy participants and patients with neurodegenerative diseases
-
Facial movement, eye tracking, hand movement data from controls and individuals with neurodevelopmental disorders
-
Physiological, behavioral and neuroimaging data from animal models of stroke
-
Neural recordings under acute or chronic stress conditions in a rodent model
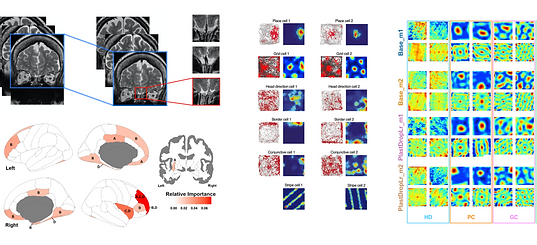
Publications
2024
Krätzig, F.*, Mei, J.*, Rebaliha, M., Rampanjato, Z., Ranaivoson, R., Razafinjato, J., De Neve, J. W., Franke, M., Muller, N., & Emmrich, J. V. (2024). Proportion of foetal and placental abnormalities in Madagascar: a cross-sectional study of 35,919 women at public-sector primary healthcare facilities in central and southern Madagascar, 2017-2020. In press.
Meshkinnejad, R., Mei, J., Lizotte, D., & Mohsenzadeh, Y. (2024). Look-ahead selective plasticity for continual learning of visual tasks. Proceedings of UniReps: the Second Workshop on Unifying Representations in Neural Models. NeurIPS 2024.
doi.org/10.48550/arXiv.2311.01617
Mei, J., & Nagai, Y. (2024). Understanding context dependency in time perception in autism spectrum disorders: a computational study. 2024 IEEE International Conference on Development and Learning (ICDL) (pp. 1-7). IEEE.
doi.org/10.1109/ICDL61372.2024.10645025
Wen, W., Mei, J.*, Aktas, H.*, Chang. A., Takada, K., Suzuishi, Y., & Kasahara, S. (2024). Control over self and others’ face: Exploitation and exploration.
Scientific Reports 14 (1), 15473.
2023
Mei, J., Meshkinnejad, R., & Mohsenzadeh, Y. (2023). Effects of neuromodulation-inspired mechanisms on the performance of deep neural networks in a spatial learning task. iScience, 26(2).
doi.org/10.1016/j.isci.2023.106026
2022
Mei, J., Muller, E., & Ramaswamy, S. (2022). Informing deep neural networks by multiscale principles of neuromodulatory systems. Trends in Neurosciences, 45(3), 237-250.
doi.org/10.1016/j.tins.2021.12.008
Nasim, M. Q., Maiti, T., Srivastava, A., Singh, T., & Mei, J. (2022). Seismic facies analysis: a deep domain adaptation approach. IEEE Transactions on Geoscience and Remote Sensing, 60, 1-16.
doi.org/10.1109/TGRS.2022.3151883
Bussiere, N., Mei, J., Lévesque-Boissonneault, C., Blais, M., Carazo, S., Gros-Louis., F., Laforce, R., De Serres, G., Dupré, N., & Frasnelli, J. (2022). Persisting chemosensory impairments in 366 healthcare workers following COVID-19: An 11-month follow-up. Chemical Senses, 47, bjac010.
doi.org/10.1093/chemse/bjac010
Ali, J., Ngo, D. Q., Bhattacharjee, A., Maiti, T., Singh, T., & Mei, J. (2022). Depression detection: text augmentation for robustness to label noise in self-reports. Digital Humanism: A Human-Centric Approach to Digital Technologies (pp. 81-103). Cham: Springer International Publishing.
doi.org/10.1007/978-3-030-97054-3_6
Kohler, J., Mei, J., Banneke, S., Winter, Y., Endres, M. and Emmrich, J. (2022) Assessing spatial learning and memory in mice: classic radial maze versus a new animal-friendly automated radial maze allowing free access and not requiring food deprivation. Frontiers in Behavioral Neuroscience, 16, 1013624.
doi.org/10.3389/fnbeh.2022.1013624
2021
Bussiere, N., Mei, J., Lévesque-Boissonneault, C., Blais, M., Carazo, S., Gros-Louis., F., De Serres, G., Dupré, N., & Frasnelli, J. (2021). Chemosensory dysfunctions induced by COVID-19 can persist up to 7 months: A study of over 700 healthcare workers. Chemical senses, 46, bjab038.
doi.org/10.1093/chemse/bjab038
Mei, J., Desrosiers, C., & Frasnelli, J. (2021). Machine learning for the diagnosis of Parkinson's disease: a review of literature. Frontiers in aging neuroscience, 13, 633752.
doi.org/10.3389/fnagi.2021.633752
Mei, J., Tremblay, C., Stikov N., Desrosiers, C., & Frasnelli, J. (2021). Differentiation of Parkinson’s disease and non-Parkinsonian olfactory dysfunction with structural MRI data. Medical Imaging 2021: Computer-Aided Diagnosis (Vol. 11597, pp. 320-327). SPIE.
doi.org/10.1117/12.2581233
2020
Kang, W., Pineda Hernández, S., & Mei, J. (2020). Neural Mechanisms of Observational Learning: A neural working model. Frontiers in human neuroscience, 14, 609312.
doi.org/10.3389/fnhum.2020.609312
​
Tremblay, C., Mei, J., & Frasnelli, J. (2020). Olfactory bulb surroundings can help to distinguish Parkinson’s disease from non-parkinsonian olfactory dysfunction. NeuroImage: Clinical, 28, 102457.
doi.org/10.1016/j.nicl.2020.102457
Shen, J., Mei, J., Walldén, M., & Ino, F. (2020). Integrating GPU support for FreeSurfer with OpenACC. 2020 IEEE 6th International Conference on Computer and Communications (ICCC) (pp. 1622-1628). IEEE.
doi.org/10.1109/ICCC51575.2020.9345102
2019
Akgun, O. C., & Mei, J. (2019). An energy efficient time-mode digit classification neural network implementation. Philosophical Transactions of the Royal Society A, 378(2164), 20190163.
doi.org/10.1098/rsta.2019.0163
Mei, J., Kohler, J., Winter, Y., Spies, C., Endres, M., Banneke, S., & Emmrich, J. V. (2019). Automated radial 8-arm maze: A voluntary and stress-free behavior test to assess spatial learning and memory in mice. Behavioural Brain Research, 381, 112352.
doi.org/10.1016/j.bbr.2019.112352
Mei, J., Banneke, S., Lips, J., Kuffner, M. T., Hoffmann, C. J., Dirnagl, U., ... & Emmrich, J. V. (2019). Refining Humane Endpoints in Mouse Models of Disease by Systematic Review and Machine Learning-Based Endpoint Definition. ALTEX: Alternatives to Animal Experimentation, 36.
doi.org/10.14573/altex.1812231
2018
Mei, J., Riedel, N., Grittner, U., Endres, M., Banneke, S., & Emmrich, J. V. (2018).
Body temperature measurement in mice during acute illness: implantable temperature transponder versus surface infrared thermometry. Scientific reports, 8(1), 3526.
doi.org/10.1038/s41598-018-22020-6
Mei, J., & Singh, T. (2018). Intra-thalamic and thalamocortical connectivity: potential implication for deep learning. Proceedings of the 1st International Workshop on Software Engineering for Cognitive Services (pp. 9-14).
doi.org/10.1145/3195555.3195556
2013
Jarmolowska, J., Turconi, M. M., Busan, P., Mei, J., & Battaglini, P. P. (2013). A multimenu system based on the P300 component as a time saving procedure for communication with a brain-computer interface. Frontiers in neuroscience, 7, 35988.
doi.org/10.3389/fnins.2013.00039